Marketing Mix Models (MMMs) are sophisticated tools that can fall prey to a phenomenon known as “overfitting.” This occurs when a model becomes excessively attuned to the training data, failing to capture the underlying causal relationships. Let’s explore this concept and its implications, drawing on insights from Mutinex’s advanced AI-driven approach.
The Overfitting Conundrum in MMM
Overfitting in marketing mix modeling happens when the marketing mix model becomes too complex, fitting noise rather than signal in the unseen data. This can lead to inaccurate interpretations of marketing campaigns, misleading results, and potentially costly decisions when applied to real-world marketing strategies.
Causes of Overfitting:
- Excessive Variables: Including too many independent variables can cause the model to latch onto random fluctuations.
- Dummy Variable Abuse: Using meaningless dummy variables to artificially improve model fit metrics.
Mutinex’s AI model addresses these issues by employing advanced feature selection techniques and regularisation methods to prevent overfitting.
Deceptive Nature of Traditional Metrics
Many marketers rely on in-sample R-squared as a measure of model quality. However, this metric can be misleading:
- High R-squared ? Good Model: A high R-squared merely indicates how well the model fits the training data, not its predictive accuracy.
- Artificial Improvement: Adding variables, even random ones, can increase R-squared without improving the model’s actual performance.
Other metrics like Mean Absolute Percentage Error (MAPE) and Root Mean Squared Error (RMSE) can also be deceptive, as they tend to improve with added complexity without necessarily enhancing the model’s predictive power.
Mutinex’s approach goes beyond these traditional metrics, utilizing ensemble learning and cross-validation techniques to ensure robust model performance.
Validating MMMs: The Power of Out-of-Sample Testing
To truly assess an MMM’s effectiveness, out-of-sample validation using training and validation sets is crucial. This ensures that the model evaluation reflects real-world conditions.
Implementing Out-of-Sample Validation:
- Train the model on historical data up to a certain point.
- Use the model to predict the following period.
- Compare predictions with actual results to assess forecast accuracy.
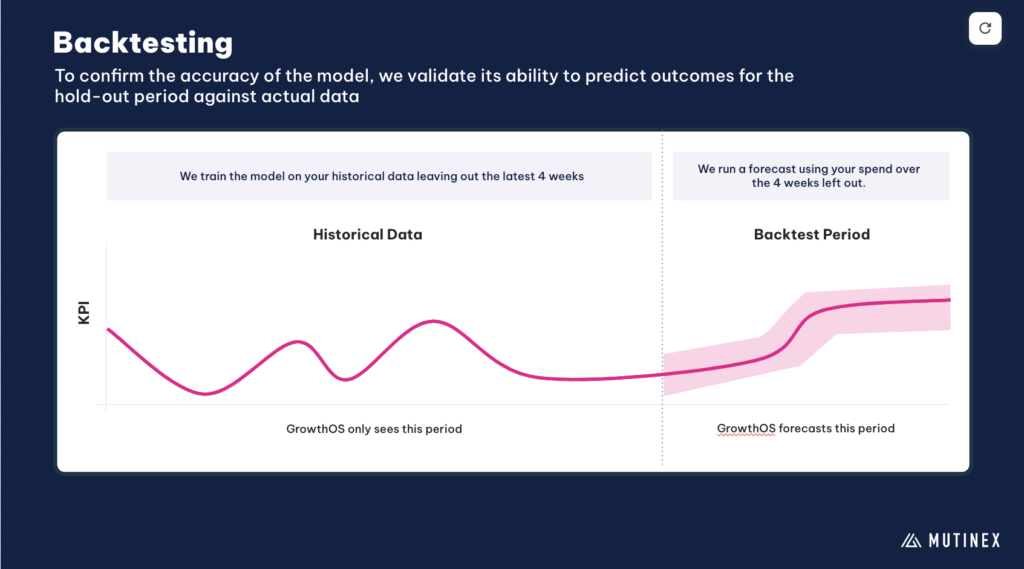
This approach is often referred to as “holdout forecast accuracy”. It’s a key component of the model’s predictive capabilities when underpinned by a strong understanding of which factors drive sales into the future.
Mutinex leverages its AI capabilities to perform continuous out-of-sample validation, ensuring that models remain accurate and relevant over time while enhancing the model’s ability to adapt to evolving market trends.
Mutinex’s AI-Driven Approach to MMM
Mutinex’s generalized AI model takes MMM to the next level by:
- Dynamic Model Selection: The system adapts to changing market conditions by selecting the most appropriate architecture from its suite of advanced machine learning models.
- Continuous Learning: Mutinex’s AI constantly updates its knowledge base, improving its ability to detect and prevent overfitting.
By combining these advanced machine learning techniques with traditional marketing expertise, Mutinex offers a robust solution to the challenges of marketing mix modeling.
The Upshot:
- Overfitting in MMM can lead to inaccurate models and poor marketing decisions.
- Traditional metrics like in-sample R-squared can be misleading and promote overfitting.
- Out-of-sample validation is essential for assessing true model performance.
- Mutinex’s AI-driven approach addresses overfitting through advanced techniques and continuous learning.
By understanding these concepts and leveraging cutting-edge AI solutions like Mutinex, marketers can develop more accurate and reliable marketing mix models, leading to better-informed decisions and improved ROI, resulting in successful business outcomes.